2024 年 5 月 19 日
Abstract
Selecting players armed with unique abilities to collaborate with is the key to crafting an unbeatable strategy while on the field, navigating a quest, or in the office. In the realm of standardized clinical trial data, not only can this break down departmental siloes, but it can also enhance the quality of study data, leading to the availability of more effective, efficient treatments earlier.
Historically, due to the timing and content of SDTM validation, many organizations enlisted SDTM programmers with unraveling issues in these reports. However, there are some rules that require tracing SDTM data back to raw data or data collection to optimize decision-making. Seamlessly integrating data managers into this process can help slash time to resolution of these types of issues as well as boost overall data quality.
This poster will provide important considerations and guidance on weaving data managers into the SDTM validation process. Unveiling different types of workflows will illustrate how to level up your approach to resolving validation issues. Curating appropriate FDA validation rules along with detailed examples will showcase how these are best served by the unique positioning of data managers. Lastly, suggested training as well as ideas to power-up your data managers will equip you to battle issues at the source and conquer those data demons.
Introduction to SDTM validation
Within many organizations, SDTM programmers have been tasked with decoding and researching issues found in the validation reports that assess the conformance of SDTM data sets. This sequence of events was implemented because validation of those data sets cannot logically be performed until after their creation. Because these programmers are often intimately involved in creating the mapping specifications using the aCRF, or creating both the aCRF and mapping specs, they become familiar with the origin of the raw data, as well as the transformations used to populate the variables in the resulting data sets. However, SDTM programmers are generally not involved in the data collection process, including creating the forms for data collection, or in the review of raw data; data managers are primarily responsible for those tasks.
This poster and paper will highlight how data managers are uniquely positioned to aid in the research and resolution of SDTM validation issues. A phased approach to integrating data managers directly within the SDTM validation process will be discussed, as well as examples of curating specific validation rules for data management to respond to as the first line of response. Details for appropriate training will be discussed and we’ll strategize how to unlock the full potential of this implementation.
Choose your player
A myriad of contenders are involved over the lifecycle of a clinical trial; one of the most important players is the role of the data manager. Data managers are often involved in several different stages of running the trial, from protocol development to database lock, and act as guardians of data collection and quality. Due to the fundamental association of the data manager’s role with the collected data itself, the data manager must form deep knowledge of the protocol, the data required to be collected for analysis, and how specific data collection should occur. They become well-versed in form design, the use of conditional fields and skip logic, and the type of data that is expected to be entered within each field. They’re also able to implement data quality checks at the data collection level, monitor the information entering the database in real time, and communicate directly with the sites if any issues arise.
These capabilities are advantageous for exploring and resolving data-related challenges, whether the data are in their raw or standardized format. Familiarity with data collection and the collected data enables data managers to quickly assess data issues.
Know the rules of the game
The first step to integrating data managers directly into the SDTM validation reporting process is to create a list of rules from the validator that would be appropriate for data managers to oversee. Although there will be some variability across studies as to which issues the data itself has been attributed as the root cause of the issue, looking across a collection of past clinical study data reviewer’s guides (cSDRGs) for studies previously completed by your organization will allow you to identify patterns of issues with this attribution. In section 4.2 of the cSDRG, the explanations can help determine if the issue has been deemed to stem from some aspect of the data collected. Similarly, since section 4.2 of the cSDRG is created using the validation report run on SDTM data sets, even a sampling of different SDTM validation reports from different studies that include notes detailing the cause of the underlying issues could be used to categorize these issues and compile this list. Also, simply reviewing the list of SDTM validation rules executed by your company’s validator, or the FDA validator rules themselves, and identifying the ones most likely to attribute the raw data as the source of the issue could lead to a win for this first level.
Walkthrough
Many data quality checks can be found within the FDA validator rules. For instance, “SD1332: AEOUT=NOT RECOVERED/NOT RESOLVED, but an end date is provided” is an issue that occurs when the data for the outcome of the adverse event and the end date of that event appear contradictory. In many cases, this occurs when the form has been completed indicating that the outcome of the adverse event was “NOT RECOVERED/NOT RESOLVED” and an end date for the AE has been recorded. The data manager will be able to quickly identify the form used to collect data for the adverse event and check the value for the two variables in the raw data to assess whether the site has entered discrepant information. If they find that an error exists, they can immediately query the site or request implementation of a data quality check at the form level for these fields.
However, in some cases, the protocol will require that an end date is recorded for all ongoing AEs at the time of the subject’s death. In this case, the outcome will still appear to conflict with the end date, but the issue can be explained by knowledge of the protocol that the data manager will possess.
Another data quality check contained in the FDA validator rules is “SD1279: ECDOSTXT is null when ECDOSE is null and ECOCCUR does not equal ‘N’”. This issue will be present when the dose for a study treatment is blank, but it is not reported in that record that the study treatment did not occur. In this example, the data manager will again quickly be able to identify the form that collects study treatment data and will be familiar with the form design. They can then determine if the form has been completed in such a way that the value of the dose should have been collected.
Using the data format of the field for the dose, they can determine if ECDOSTXT and ECDOSE would be populated using the value from a single field (e.g., if the dosing field only collects numeric values, then ECDOSTXT would not be populated using that field), or if separate fields would be used to collect values for each of these variables. If it was reported that study treatment was given, but no dosing information is present that would populate ECDOSE or ECDOSTXT, they would be able to query the site to obtain the dosing information and ensure a form-level check is added to the dosing information field(s) when study treatment has been reported to occur. If it was reported that study treatment was not given, they would need to escalate the issue to an SDTM programmer so that ECOCCUR was correctly populated as “N”. Alternately, if the data manager is unable to assess whether the study treatment occurred due to lack of information on the form, they would be able to query the site to address the issue.
Data managers could also handle SDTM validation issues of greater complexity. For instance, in “SD0080: AE start date is after the latest Disposition date”, there are two SDTM domains involved and a requirement that the latest disposition date for the subject is known, which will likely involve multiple forms. However, the data manager will be able to quickly locate the Adverse Event form and verify if the start date for the event seems to have been reported correctly (e.g., the year seems relevant to the collection period). It may be more time consuming to identify the latest disposition date for the subject, however, the data manager will be aware of which forms collect this type of information and can find the most recent date reported for the subject within the various forms. They may even be aware of reports built from the raw data to show this type of information at-a-glance and would be able to find the latest disposition date much more quickly this way than looking directly at each raw dataset to find the latest date.
Many times, the issue can be identified as having been a data entry error, but in some cases, it arises from the timing of validation (i.e., prior to the subject going off study) or may be attributed to protocol requirements. For example, this issue will appear in cases where the participant is still active on study at the time the AE begins and the only disposition start date reported is the date of informed consent. In this case, the issue would be expected to be resolved once the participant discontinues or completes the study, provided that the date of that disposition event follows the start of the AE. However, in some studies, adverse events are collected and reported after the participant discontinues or completes the study, which is specified by the protocol. Again, this would be an advantage that the data manager would have from knowing explicit information contained in the protocol.
Of course, there are issues flagged by the FDA validator rules that may not be best suited to be handled by data management as front-line support. An example of one of these rules could be “SD0036: Missing value for LBSTRESC when LBORRES is provided”, particularly when laboratory test results are collected only via eCRF. This issue occurs when LBORRES has been populated, but no value exists in the variable LBSTRESC. Although, in this case, the value for LBORRES is being collected via the eCRF, the value for LBSTRESC is generally mapped using the value of LBORRES so that it is standardized. For instance, LBORRES could contain “NEG”, “NEGATIVE”, or “NONE” which effectively have the same meaning. When mapped to LBSTRESC, “NEGATIVE” could be used for all three values of LBORRES to keep the LBSTRESC values standard and consistent. When LBSTRESC is null, but a value has been captured for LBORRES, then the derivation or assignment of the value is most likely the root cause of the issue, not the data captured, and proper resolution of this issue could only be provided by an SDTM programmer.
Beginners guide
Before data managers will be able to successfully manage the subset of data issues from the SDTM validation report, some initial training should be conducted so that they understand the basic concepts of SDTM data set creation. Since mapping raw data or annotating an aCRF is outside the purview of this mission, training does not need to be as broad or intense as for someone who is required to perform those tasks. Thus, a more targeted approach can be used as the goal is to ensure data managers can trace data found in SDTM variables back to their original data collection variables. Below is a list of concepts that would be helpful for data managers to become familiar with:
- Basics of SDTM and CDASH, if the company is using the CDASH standard
- What is a domain and what classes are used for different categories of data?
- Difference between, the importance of, and how to use:
- An eCRF
- An eCRF annotated with EDC variables
- An SDTM annotated CRF (aCRF)
- Basic mapping specification concepts (e.g., how to read a mapping spec)
- How to determine the association between domain, raw data set, and form
- What common transformations are used and how they impact the raw data value
Power-ups and cheat codes for your team
Suggested strategies to aid in addressing SDTM validation issues include managing a collection of the issues data management will take on along with steps on how to research each one with examples. Creating a list of examples with steps to take may even be an activity requested to be performed by the data manager when reviewing their first few issues to help familiarize them with the process of examining specific issues. It may also help to categorize issues by the level of complexity of the research involved to resolve them. For example, it is much more straightforward to investigate a data issue where the value of one variable is reviewed than cross-checking raw data values for two or more different variables. Then, begin assigning issues for data management to take charge of, starting with those considered least complex to investigate, and progressively moving on to more challenging ones.
Another tool that’s helpful when reviewing SDTM validation reports is a combined eCRF that contains both the EDC field annotations as well as SDTM annotations. This will make it easier for the data manager to begin associating EDC and SDTM variables with each other. If standard forms are used, they will help boost that association since fields with the same names will be consistently associated with the same SDTM variable across studies and the repetition will intrinsically aid retention.
Begin your quest
After the basics are learned, the fun can begin! But you won’t want to just throw everyone into the deep end. Beginning implementation could include pairing an SDTM validation report expert with a data manager during the first few reviews so that they can ask questions as they work through resolving the issues. Once a couple of initial rounds have been completed, weekly or bi-weekly office hours between data managers and representatives from clinical data standards and SDTM programming teams could be arranged for data managers to raise questions on any issues they need help researching. Concurrently, meetings within the data management group to share experiences resolving issues in the SDTM validation reports would help to ensure knowledge transfer within the team. Lastly, a dedicated internal Q&A page for questions on specific issues within the SDTM validation report could be instituted so that those with similar questions have a resource to autonomously find answers.
结论
Data managers should be incorporated as key players in the SDTM validation process because they possess a wide range of skills and capabilities that can greatly contribute to the goal of submitting clean, compliant data. Coupled with the unique position of their role, their direct involvement in handling SDTM validation issues can lead to decreased issue research and resolution time as well as enable better decision making faster. By choosing specific issues for data managers to address, providing necessary, targeted training, using a phased approach to delegate issues, and offering support via mentoring and open communication will establish a winning strategy destined to dominate any data discrepancies.
Acknowledgments
We would like to thank Wendy Young and Liz Hamilton for working with us to help create the associated poster. Their artistic skill and help with editing truly assisted with bringing our vision to life. Ready to start creating your SDTM data sets?
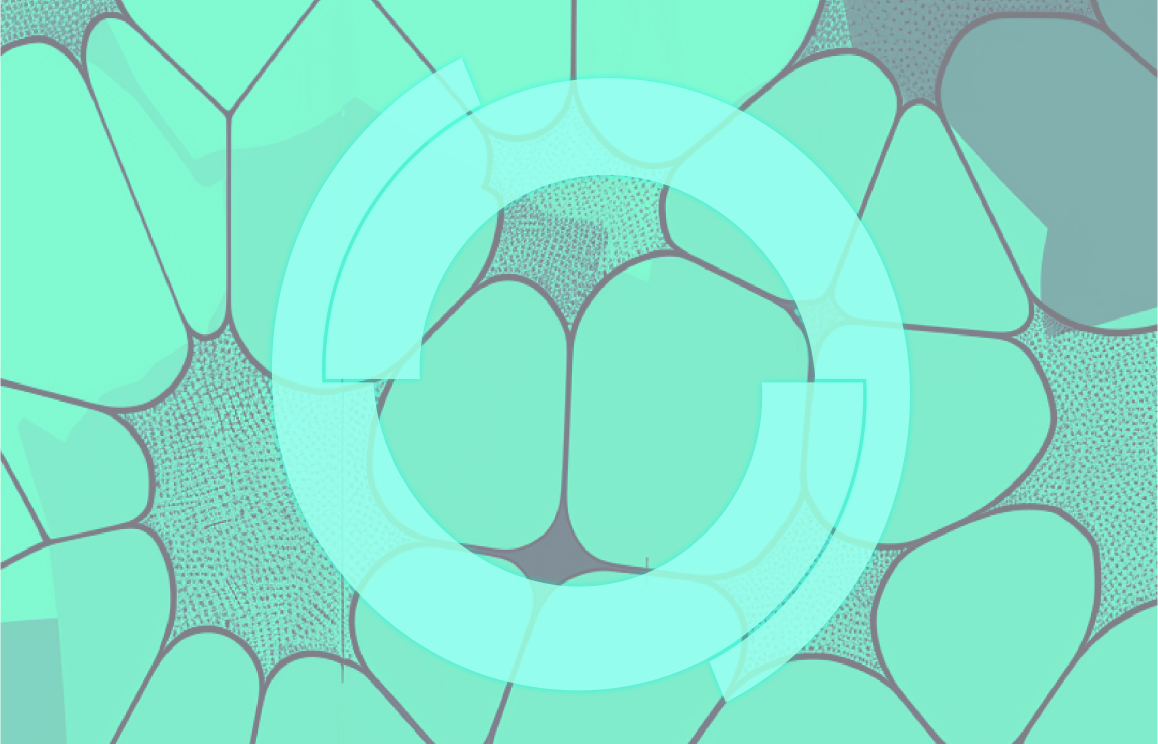
A Quick Guide to SDTM Dataset Creation
Our guide can help you better understand SDTM datasets and get started quick with SDTM dataset creation.
参考文献
[1] Food and Drug Administration. 2022, December. “FDA Validator Rules v1.6 December 2022.”Accessed 2024 年 2 月 15 日. https://www.fda.gov/media/103587/download
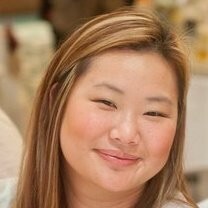
Principal Consultant
Julie Ann Hood is a Principal Consultant at Pinnacle 21. She received her Master’s Degree in Psychology from the University at Buffalo where she completed the Behavioral Neuroscience program and embarked on her drug development journey in pre-clinical research at the Research Institute on Addictions. After transitioning into clinical research as a Data Manager and being introduced to CDISC, she gained over 10 years of experience consulting on submission readiness and clinical data standards development.
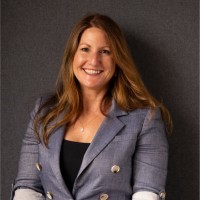
Subject Matter Expert and User Advocate
Jen Manzi is a Subject Matter Expert and User Advocate at Pinnacle 21. She has over 20 years of Pharma/Life Sciences industry experience in Clinical Trials and Safety Data Management. Jen has held various roles within these areas, including eCRF Programmer, SDTM Delivery Lead, Product Owner and Programmer of Batch Processes, Vendor Relationship Manager, Program and Process Improvement Manager, and Validation Lead.
联系我们